What Does AI Know About Having A Ball?
Jaspreet Bindra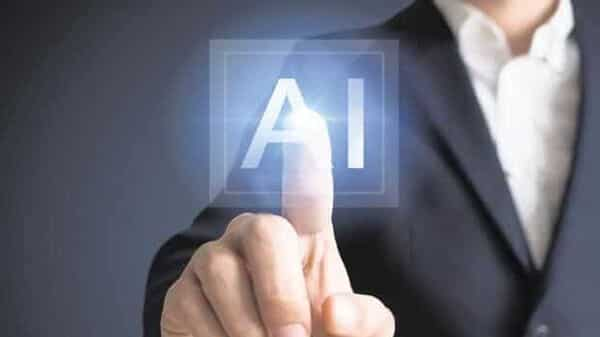
I typed ‘gorilla in a grass skirt having a ball’ into a search-like box on craiyon.com, and the site promptly threw up a set of images of a good-looking gorilla, wearing a very Hawaiian grass skirt. But its version of having a ball was not to have a party, but to hold a large colorful ball in its arms. And therein lies the rub. While DALL-E Mini, the original name of Craiyon is absolutely fantastic, it is still got some way to go. It is the open source, free and a slightly attenuated version of the mother neural network programme DALL-E 2, created by OpenAI. DALL-E, along with Imagen released by Google Brain to one-up OpenAI, are the latest AI LLMS (Large Language Models), which are stretching the boundaries of what AI can do.
In August 2020, I wrote about the stunning story-telling prowess of another LLM, GPT3. The Generative Pre-trained Transformer Ver 3, I wrote, is being heralded as the first step towards the holy grail of AGI (Artificial General Intelligence), where a machine that has the capacity to understand or learn any intellectual task that a human being can. GPT has been trained on a massive body of text, mined for statistical regularities or parameters or connections between the different nodes in its neural network. The scale is gargantuan, with it having 175bn parameters, all of Wikipedia comprises just 0.6 percent of its training data! GPT-3 was developed by OpenAI too, and with DALL-E they took this to another level. OpenAI took a 12 billion parameters version of the GPT-3 model and trained it to interpret natural language inputs and generate images corresponding to it; thus literally ‘swapping texts for pixels’. So, if the text prompt was ‘an astronaut riding a yellow horse near Saturn’, the program would break up this sentence into segments of information, find an image closest to it and then synthesise all of it to show an astronaut sitting on a horse against a starry sky with Saturn hovering in the background. A sister model called CLIP (Contrastive Language Image Pretraining) would then rank the outputs created based on certain parameters and curate the ones thatwith the best quality to show you. The model was trained on large numbers of photos either scrapped from the internet or acquired from licensed sources.
This is not new. Neural network based image generation has been prevalent since the beginning of this century. What is new with DALL -E has been how it has been able to do so from natural language prompts, the way you and I would ask a question, and to produce very meaningful outputs. To truly understand the magic, I would actually ask you to go to Craiyon and play with it on your own. You might feel a bit like what Marcelo Rinesi, the CTO of Institute of Ethics and Emerging Technologies, did when he watched Jurassic Park. In WIRED magazine, he remembers that the dinosaurs looked so realistic that they “permanently shifted people’s perception of what’s possible.”. After playing around with DALL-E 2, thinks that “AI might be on the verge of its own Jurassic Park Moment.” As you play around with DALL-E 2, or Imagen, you will find it to be a powerful tool, but as Rinesi says, “it does nothing a skilled illustrator couldn’t with Photoshop and some time. The major difference, he says, is that DALL-E 2 changes the economics and speed of creating such imagery.”Twitter seemed to agree: “We’re living through the AI space race!” said one Twitter user commented. “The stock image industry is officially toast,” tweeted another.
These ground-breaking models do come with their own series of problems, though. An obvious one is bias, LLMs like this make it possible to ‘industrialize disinformation or customize bias’. The creators of these models know this. Imagen for example was quite blasé in its disclaimers: “While a subset of our training data was filtered to remove noise and undesirable content, such as pornographic imagery and toxic language, we also utilized LAION-400M dataset which is known to contain a wide range of inappropriate content including pornographic imagery, racist slurs, and harmful social stereotypes. “Imagen relies on text encoders trained on uncurated web-scale data, and thus inherits the social biases and limitations of large language models.” Some of you might remember the bold experiment by Microsoft with an AI chatbot called Tay, which within hours started spouting antisemitic, racial and pornographic talking points. When OpenAI, launched GPT-3, it famously said that ,“internet-trained models have internet-scale biases.” That in fact is the nub of the issue: content for the Internet is created by human beings, and a portion of it reflects the racial and gender biases of the creators themselves and reflects the same flaws in their creations. I wrote of GPT-3 as evidence that AI can be creative like humans, and DALL-E 2 seems to be another large one towards that. OpenAI was thinking similarly perhaps: DALL-E is the combination of the robot WALL-E and the creative master Salvodar Dali. But as my experiment with the gorilla having a ball goes, while it can still don a grass skirt, it will take some time for it to come to the party.